Paper Accepted to CIARP 2024!
Our paper entitled “Exploiting the Segment Anything Model (SAM) for Lung Segmentation in Chest X-ray Images” was just accepted for publication in the Iberoamerican Congress on Pattern Recognition.
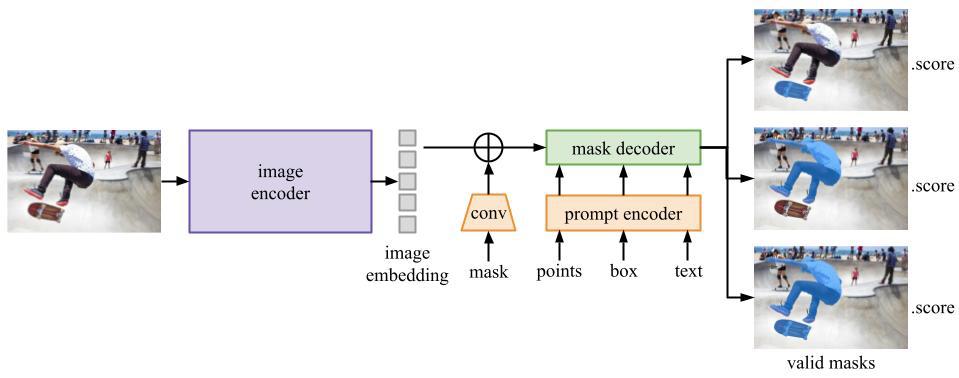
Segment Anything Model (SAM) (Kirillov et al., 2023) is a tool that, since its release in April 2023, has proven to be very promising in the task of image segmentation. Its approach involves using a variety of input prompts to identify different objects in images, such as points and bounding boxes. To predict the masks, SAM uses three components: (i) an image encoder, (ii) a prompt encoder, and (iii) a mask decoder. Additionally, the model can automatically segment anything in an image and generate multiple valid masks for ambiguous inputs, which is innovative in the field.
Given this and the immense amount of data used in its training – 11 million images and over 1 billion masks (Kirillov et al., 2023) – many researchers have recognized the potential of this technology in the medical field and have begun to investigate its effectiveness in this area. However, despite having this large volume of data in its training, there are no medical images among the domains in which SAM was trained, which makes its generalization ability moderate when it comes to this area (Huang et al., 2024, Mazurowski et al., 2023).
This study aims to advance the application of SAM in the field of medical image analysis, especially, for lung segmentation in chest X-ray images. Understanding the effectiveness of SAM in this domain is of paramount importance in the development of new technologies for the diagnosis, treatment and follow-up of lung diseases. We finetune SAM on two collections of chest X-ray images, known as the Montgomery and Shenzhen datasets (Jaeger et al., 2014). This well-established practice aims to leverage the benefits of representations previously learned on a larger database to optimize the training of a network on a smaller dataset. Our exploration also involved testing SAM across such datasets using various input prompts, like bounding boxes and individual points. The obtained results show that our finetuned SAM can perform similar to state-of-the-art approaches for lung segmentation, like U-Net (Ronneberger et al., 2015).
Do you want to know more about fine-tuning SAM for lung segmentation in chest X-ray images? Check our paper!