New Journal Paper Out!
Our paper entitled “An Edge Computing-Based Solution for Real-Time Leaf Disease Classification using Thermal Imaging” was just published in the IEEE Geoscience and Remote Sensing Letters.
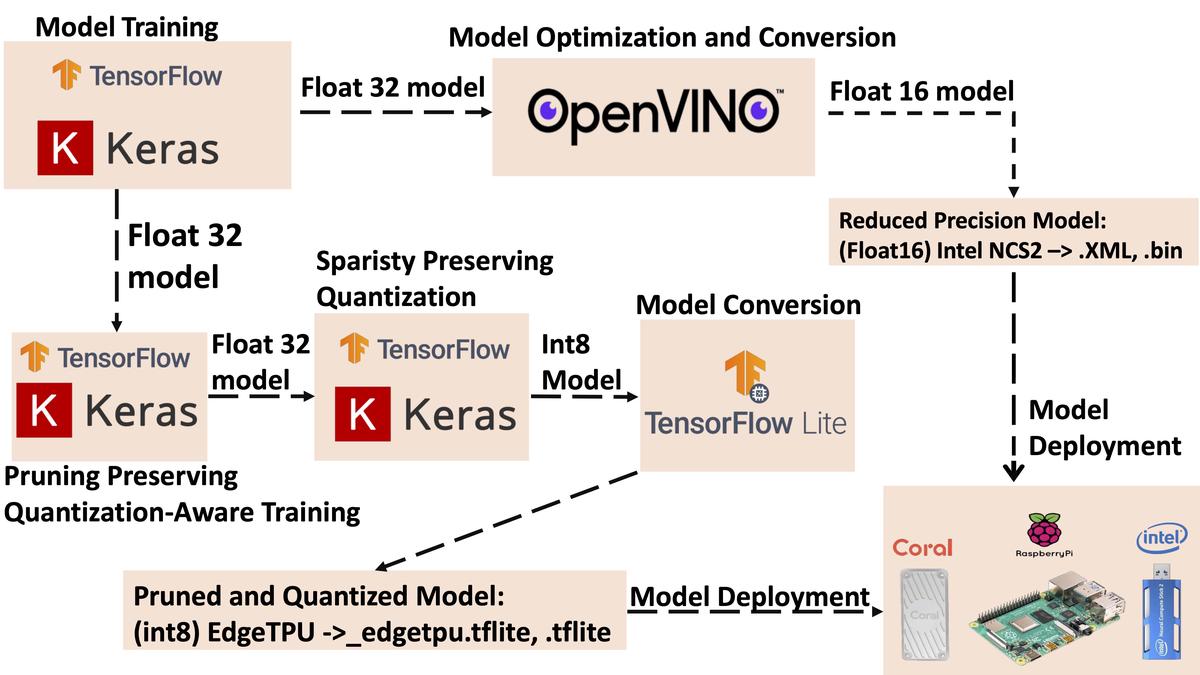
Diseases in plants caused by living entities can be classified as biotic and abiotic (Kaur et al., 2019). The primary sources of these biotic diseases are fungi, bacteria, and viruses. Conversely, abiotic diseases arise from non-living environmental factors, including hail, spring frosts, various weather conditions, exposure to chemicals, and more. These abiotic diseases, being non-infectious and non-transmissible, are generally less harmful and often preventable.
Plant diseases pose a significant threat to food security, requiring prompt detection. However, the complexity of these diseases often challenges even experienced agronomists and plant biologists, leading to potential misdiagnosis and improper treatment (Batchuluun et al., 2022). In São Paulo, Brazil, crops are particularly vulnerable to climate variability, with extreme weather events like droughts, heat, and frost further complicating agricultural productivity. To address these challenges, efficient agricultural techniques such as crop rotation, cover cropping, and water management are essential for safeguarding and enhancing productivity (Nascimento et al., 2023).
In addition, the significant reduction in crop yield is due to the inability to detect diseases early on, which invariably leads to decreased agricultural output. Consequently, the early recognition and examination of crop diseases are essential for maintaining crop quality. With recent advancements in computing speed and power, the use of large datasets has enhanced the efficiency of these systems (Paymode and Malode, 2022). Therefore, the development of an automated process for leaf disease detection can also help with the reduction of agrochemicals and pesticide.
Most research on leaf disease classification has focused on visible images (Khan et al., 2022, González-Huitrón et al., 2021, Shahidur Harun Rumy et al., 2021, Brahimi et al., 2017), which are sensitive to light conditions and may underperform without daylight. In contrast, thermal imaging, with its potential for overcoming these limitations, has been widely used in studies on environmental stress, crop yield, and seed vigor (Wen et al., 2023).
Moreover, the use of traditional cloud infrastructures approaches for image classification poses a latency challenge between an edge device and the cloud (Varghese et al., 2016), which is not feasible for real-time image classification. In this context, edge computing is a paradigm that facilitates computation near data sources, allowing processing at the edge layer instead of in the cloud. This approach is particularly advantageous when handling numerous distributed data sources where cloud computing may not be feasible (Zhang et al., 2019).
Therefore, in this study we leverage the use of an embedded device such as the Raspberry Pi 4B as an edge device and perform aggressive model compression by means of pruning-quantization aware training (PQAT) in addition of hardware acceleration devices such as the Edge TPU and Intel NCS2 to perform real-time image classification of leaf diseases using thermal camera.
Are you looking for a robust dataset for real-time classification of leaf diseases using thermal imaging? Check our paper!