Video Understanding through Deep Learning with Minimal Human Supervision
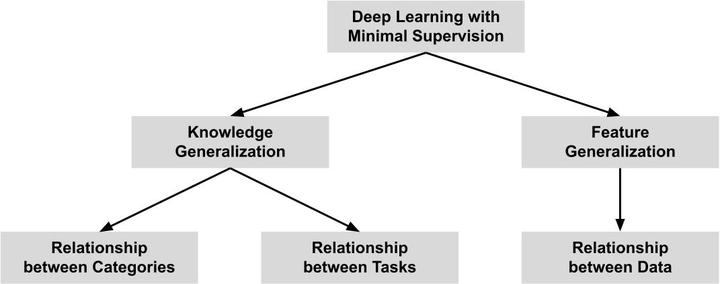
Digital videos have become the medium of choice for a growing number of people communicating via Internet and their mobile devices. Over the past decade, world has witnessed an explosive growth in the amount of video data fostered by astonishing technological developments. In this scenario, there is a growing demand for efficient systems to reduce the work and information overload for people. Making efficient use of video content requires the development of intelligent tools capable to understand videos in a similar way as humans do. This has been the goal of a quickly evolving research area known as video understanding. Last years, thanks to its great learning capacity from data exposure, deep learning has led to remarkable progress in video understanding. Largely, this is due to the availability of large amounts of labeled data that has contributed to the development of models with extraordinary inference capabilities. Acquiring and annotating a desirable amount of data to satisfy these models is often a hard task for many application domains, requiring overwhelming human effort and, sometimes, specific expertise. This reliance on exhaustive labeling is a key limitation to deploying video understanding systems in the real world. Although deep learning models excel in many video understanding tasks, occasionally beating humans, their generalization ability is poor, only performing tasks they are trained for. The ability to adapt to novel scenarios is the hallmark of human intelligence. Motivated by such aspects, this research proposal aims to investigate methods to improve the generalization ability of deep learning, enabling to perform video understanding with minimal human supervision. The main scientific contribution will be a new and more sustainable pipeline for deep learning applications, requiring tailored human knowledge to disambiguate only critical decisions. For this, we intend to advance the state of the art in model generalization, aiming to learn with most informative data and put the human in the loop in a more effective manner. Finally, the results of this research proposal are also meant to enable companies to put AI into their products or production systems reducing the need for experienced operator knowledge for data annotation.
Funding agency | FAPESP |
Support type | Regular Research Grants |
Grant number | 2023/17577-0 |
Title | Video Understanding through Deep Learning with Minimal Human Supervision |
Duration | September 01, 2024 - August 31, 2026 |
Status | In Progress |